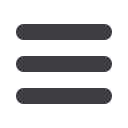
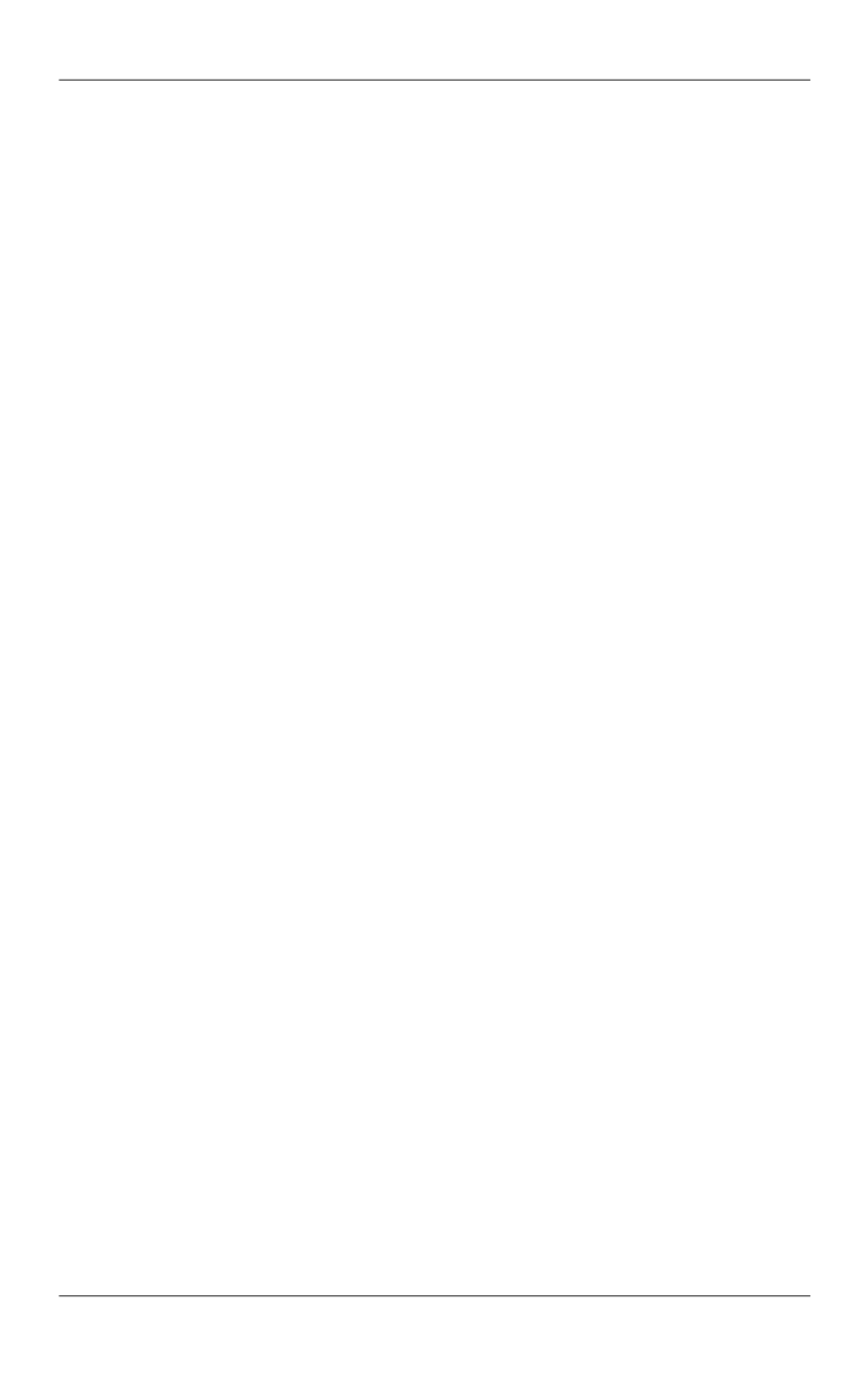
Предсказание атрибутов профиля пользователя социальной сети…
ISSN 0236-3933. Вестник МГТУ им. Н.Э. Баумана. Сер. Приборостроение. 2017. № 2
73
2.
Li R., Wang C., Chang K. C.-C.
User profiling in an ego network: co-profiling attributes and rela-
tionships // Proc. 23rd Int. Conf. on World Wide Web. WWW '14. New York: ACM, 2014.
P. 819–830. URL:
http://wwwconference.org/proceedings/www2014/proceedings/p819.pdf3.
Dong Y., Yang Y., Tang J., Yang Y., Chawla N.V.
Inferring user demographics and social strate-
gies in mobile social networks // Proc. 20th ACM SIGKDD Int. Conf. on Knowledge Discovery and
Data Mining. KDD '14. New York: ACM, 2014. P. 15–24.
4.
Анализ
социальных сетей: методы и приложения / А. Коршунов, И. Белобородов, Н. Бу-
зун, В. Аванесов и др. // Труды Института системного программирования РАН. 2014. Т. 26.
№ 1. С. 439–456.
URL:
http://cyberleninka.ru/article/n/analiz-sotsialnyh-setey-metody-i-prilozheniya5.
Mislove A., Viswanath B., Gummadi K.P., Druschel P.
You are who you know: inferring user pro-
files in online social networks // Proc. 3d ACM Int. Conf. on Web Search and Data Mining.
WSDM '10. New York: ACM. 2010. P. 251–260.
6.
Chaabane A., Acs G., Kaafar M.
You are what you like! Information leakage through users’ inte-
rests // Proc. Annual Network and Distributed System Security Symposium. 2012.
7.
Kosinski M., Stillwell D., Graepel T.
Private traits and attributes are predictable from digital rec-
ords of human behavior // Proc. of the National Academy of Sciences. 2013. Vol. 110. No. 15.
P. 5802–5805. DOI: 10.1073/pnas.1218772110 URL:
http://www.pnas.org/content/110/15/5802.full8.
Dougnon R.Y., Fournier-Viger P., Nkambou R.
Advances in artificial intelligence // Proc. 28th
Canadian Conf. on Artificial Intelligence. Canada: Springer International Publishing, 2015.
P. 84–99.
9.
Yang J., Leskovec J.
Community-affiliation graph model for overlapping network community
detection // 12th IEEE Int. Conf. on Data Mining, ICDM 2012. 2012. P. 1170–1175.
DOI: 10.1109/ICDM.2012.139 URL:
http://ieeexplore.ieee.org/document/641373410.
Чесноков В.О.
Выделение пересекающихся сообществ в социальных графах по мажори-
тарному признаку соседей // ЛОМОНОСОВ — 2016. XХIII Международная научная конфе-
ренция студентов, аспирантов и молодых ученых. 2016. М.: МАКС Пресс, 2016. С. 49–51.
11. Clauset A., Newman M.E.J. Moore C.
Finding community structure in very large networks //
Phys. Rev. E. 2004. Vol. 70. No. 6. P. 1–6. DOI: 10.1103/PhysRevE.70.066111
URL:
http://journals.aps.org/pre/abstract/10.1103/PhysRevE.70.06611112.
Rosvall M., Bergstrom C.T.
Maps of random walks on complex networks reveal community
structure // Proc. of the National Academy of Sciences. 2008. Vol. 105. No. 4. P. 1118–1123.
DOI: 10.1073/pnas.0706851105 URL:
http://www.pnas.org/content/105/4/1118.full13.
Yang J., Leskovec J.
Overlapping community detection at scale: a nonnegative matrix factoriza-
tion approach // Proc. of the 6th ACM Int. Conf. on Web Search and Data Mining. WSDM '13.
New York, 2013. P. 587–596. DOI: 10.1145/2433396.2433471
URL:
http://dl.acm.org/citation.cfm?doid=2433396.243347114.
Yang J., McAuley J.J., Leskovec J.
Community detection in networks with node attributes // 2013
IEEE 13th Int. Conf. on Data Mining. 2013. P. 1151–1156.
15.
Leskovec J., Krevl A.
SNAP datasets: stanford large network dataset collection // Stanford
Network Analysis Project: веб-сайт. URL:
https://snap.stanford.edu/data(дата обращения:
12.01.2017).
16.
Чесноков В.О., Ключарёв П.Г.
Выделение сообществ в социальных графах по множеству
признаков с частичной информацией // Наука и образование: научное издание МГТУ
им. Н.Э. Баумана. Электрон. журн. 2015. № 9. С. 188–199. DOI: 10.7463/0915.0811704